top of page
Jasmine Yike Wang
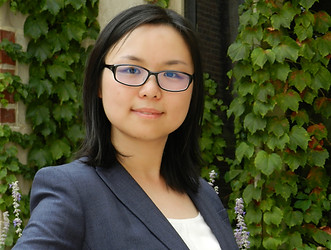
My research focuses on two interrelated areas. The first area is econometrics, with primary interests in latent variable modeling and panel data. I bring the statistical and computational insights from machine learning into the causal inference framework in economics (one example is my job market paper). The second area is to understand individual behaviors in modern digital economy that have substantial policy implications to online platforms and policy makers. This area involves massive online datasets, and applies reduced form and structural estimation, random experiments, machine learning techniques, as well as new results from my econometrics research (my working paper is an example; please see my research statement for more details).
I am a Ph.D. Candidate in the Department of Economics at the University of Chicago. I will be available for interviews at the 2019 ASSA Annual Meeting.
CV
research
WORKING PAPERS:
​
-
"Panel Data with High-Dimensional Factors: Inference on Treatment Effects with an Application to Sampled Networks" (Job Market Paper, Under Revision)
​​​
Abstract: Factor models are widely used in economics to capture unobserved aggregate shocks and individual reactions to the shocks. While the existing literature focuses on models with a small and fixed number of factors, we develop a new method in this study to allow for a large and growing number of factors under a sparsity assumption on the factor loadings. We call the new approach the High-Dimensional Interactive Fixed Effects (HD-IFE) estimator. We provide conditions under which the new estimator is consistent and asymptotically normal. We apply the HD-IFE estimator to the estimation of peer-effects models when the researcher only observes a sample of individuals and the connections among them. In this setting, missing nodes and connections create an endogeneity problem for standard regression analysis, whereas the new estimator provides consistent peer-effects estimates. The sparsity condition of the new estimator assumes that each individual is only affected by a small subset of factors. This is a plausible condition in our empirical application when network connections are sparse, as we observe in a wide range of real-world networks. Monte Carlo simulations demonstrate that when the data generating process contains a large number of factors, the HD-IFE estimator recovers the treatment-effects coefficients and latent factors well, whereas the existing low-dimensional methods in the literature underperform. Empirically, we apply the peer-effects model to examine the existence of tacit collusion on price in the Houston gasoline retail market, for which we obtain different findings by using the new estimator and the low-dimensional ones.
​
-
"Consumer Online Search with Partially Revealed Information" (with Chris Gu), Revision Invited by Management Science (New Version Coming Soon)
​​
Abstract: Modern day search platforms, such as Google or Expedia, generally have two layers of information presentation. The outer layer displays the collection of search results with attributes selected by platforms, and consumers click on a product to reveal all its attributes in the inner layer. The amount of information revealed in the outer layer affects the consumer search costs and the probability of finding a match. To address the managerial question of optimal information revelation for the first time, we create an information complexity measure of the outer layer and study the consumer search process for information at the expense of time and cognitive cost. We leverage a unique and rich panel dataset tracking consumer search behaviors at a large Online Travel Agency (OTA) that allows us to identify the associated cost and the information acquired at each consumer search step. We find that cognitive cost is a major component of search cost, and the share of loading time cost in the overall search costs is much smaller. By varying the information revealed in the outer layer, we find that price revelation shifts consumer search behavior most dramatically compared to the other product attributes. We propose information layouts that Pareto-improve both revenue and consumer welfare for the OTA.
​​
RESEARCH IN PROGRESS:
​​
-
"Numerical Likelihood Estimator for Sequential Search Models" (with Chris Gu)
​​
Abstract: Sequential search model likelihood is a high dimensional object with constraints implied by the observed consumer behavior. Traditional way of computing such likelihood uses simulated method with fixed sampling size. This leads to inaccurate and slow estimator, and limits the complexity of the model class that can be used. Furthermore, as the number of searches grows in a purchase session, the dimension of the probability space grows, and hence the number of simulation has to grow as well, putting more pressure on the simulation based method. We characterize the probability space and develop a numerical estimator that computes the exact likelihood without simulation. As a result, our method resolves the problem of simulation-based method, and saves the computation time by factor of thousands in typical short search panel context, or more when consumers have longer search panel.
teaching
TEACHING ASSISTANT:
​
The University of Chicago, Ph.D. Courses:
​​
-
Software Engineering for Economists, Professor Philipp Eisenhauer
-
Software Engineering Bootcamp, Professor Philipp Eisenhauer
-
Analysis of Microeconomic Data, Professor Dan Black
-
The Origins and Consequences of Inequality in Capabilities, Professor James Heckman
-
Social Interactions and Inequality, Professor Steven Durlauf
​​
The University of Chicago, Undergraduate Courses:
​
-
Women, Work and Property Rights, Professor Grace Tsiang
reference
REFERENCES:
​​
-
Professor Stéphane Bonhomme (Chair)
​ sbonhomme@uchicago.edu​
​​
-
Professor Chris Hansen
​ Christian.Hansen@chicagobooth.edu
​​
-
Professor Elena Manresa
​ elena.manresa@nyu.edu
bottom of page